The current pandemic and global lockdown have not only had a profound impact on people’s lives and businesses – productionised machine learning systems that predict human behaviour are also victims of COVID-19.
Examples include models predicting sales and demand on high streets, in supermarkets or in the energy sector. Predictive models are used extensively in customer relationship management, specifically for customer profiling, segmentation and retention. Inaccurate demand forecasts can be extremely expensive, for example in lost revenue and reputational damage if under-predicting demand, or in waste due to over-predicting demand. These fine-tuned and well-validated models are now worthless.
Human behaviour changed overnight when social distancing and lockdown measures were imposed across the world. This is an unprecedented event that is entirely outside the space in which machine learning systems will have been trained on. Machine learning relies on identifying patterns in historical data, but when there is nothing in the history to match the current situation, trained predictive models switch from interpolation to extrapolation. Extrapolation is difficult, very difficult, even for the best trained and validated predictive models, since, unlike interpolation, it requires a good understanding of the underlying processes to determine how the system should behave under unseen circumstances.
Machine learning is often criticised for not giving insight into why specific predictions are made. Widely used black-box models (such as deep neural networks and random forests) are specifically problematic when the models are extrapolating because we often do not understand why the predictions are wrong. With simpler and more transparent techniques (regression models) you may sacrifice some of the predictive power when the models are working on data very similar to what they have been trained on, but you gain the power of being able to explain how your models have made every prediction. The latter is important when the model is extrapolating: the predictions may be wrong, but you can understand why and can act accordingly.
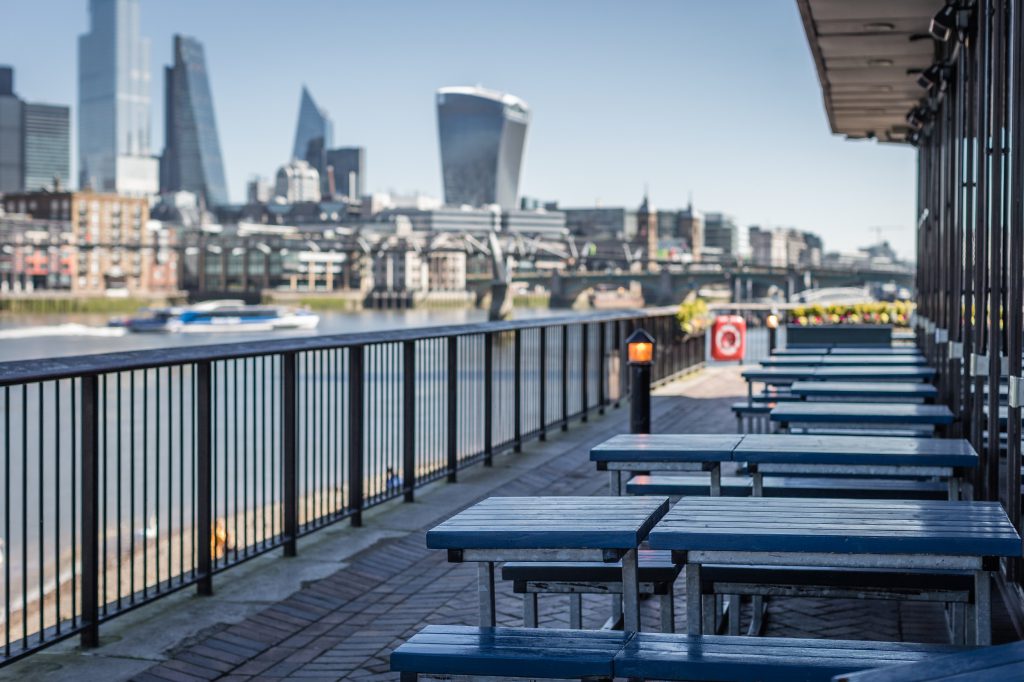
Explainable AI is an approach which promotes transparency and interpretability of AI models; with each prediction getting a score for each predictor variable representing how much they have contributed to a specific prediction or using transparent surrogate models to approximate trained machine learning models. Explainable AI will get a boost in the wake of the pandemic, when data scientists are required to not only produce models with good cross-validation reports and predictive power, but also be able to explain the predictions made in extreme situations.
Historical data is largely irrelevant now the world is in lockdown for predicting the immediate future. But looking forwards to when the restrictions will be lifted and the world will gradually return to its normal state, there will be a significant chunk of the historical data that will be polluted due to COVID-19 and the impact the lockdown has had on our behaviours, on our daily lives and on the economy. Data scientists will feel the effects of COVID-19 for years to come as they will have to deal with data collected during the pandemic and ensure that it does not corrupt their predictive models.
There are solutions around this. A simple but crude solution is to discard all data influenced by COVID-19. However, this leaves a large gap in the data which will be undesirable for some models (models predicting demand relative to the previous year’s demand for example), while other models will struggle when the state of the world on either side of the gap is very different. Defining the period to exclude may also not be clear-cut with a gradual reopening of society and will differ between countries.
More sophisticated and higher yielding solutions to this problem include augmenting the historical data with relevant information capturing the lockdown period and the forthcoming gradual reopening. In other cases, parts of the historical data will not be useful at all after the pandemic because business priorities have changed, and organisations will work towards a different set of KPIs that favour more resilience and robustness than in the past. In this instance, entirely new sources of data must be collected and brought into the predictive models.
Predictive models created by blindly applying machine learning techniques to historical data of human behaviour have been a victim of COVID-19. But augmenting data and explainable AI, provides data scientists with tools to create powerful and robust predictive models for the new norm.